[Background]
1. Degradation
ImageNet Challenging에서 depth를 늘리는 것만으로도 성능이 향상됨을 보여주었으나, 실제로는 depth는 어느정도 상승하다가 일정 시점을 넘어서면 vanishing/exploding gradient 문제를 발생시킴
이와 같이 depth가 깊은 상태에서 학습을 많이 진행한 경우 weight들의 분포가 균등하지 않고 역전파시 기울기가 충분하지 않아 안정적인 학습을 할 수 없게 하는 문제를 degradation 문제라고 함
[Related Work]
1. Blind Image SR
- degradation estimation
- establishing more realistic training data
위와 관련한 연구의 paradigm은 아래와 같음
- degradation model parameters estimating
- applies non-blind SR methods
하지만, text image들은 specific하고 semantic한 structures을 가지므로, 본 논문에서는 정교하게 설계된 deradation 모델만으로는 좋은 복원 성능을 얻을 수 없음을 보여줌
2. Text Image SR
- 전통적인 방법들
- 1. maximum a posterior (MAP)
- 2. Bayesian framework
- 최근 방법들
- 1. SRCNN
- 2. GAN기반 SR
- 3. RealSR
- 4. SRRAW
- 5. Transformer기반 SR
3. Generative Structure Prior in Image SR
- StyleGAN with codebook
- W space : controlling font style
[Main Contribution]
1. Blind SR tasks using structure prior
2. single constant를 discrete codes로 대체함으로써 StyleGAN을 재구성함
3. prior를 정확하게 검색하기 위해, LR 입력에서 codebook의 font styles, character bounding boxes 및 index를 함께 예측하는 Transformer 기반 인코더 제안
[Proposed Method]
- 기존 연구 : 주로 얼굴 복원을 위해 사전을 사용
- 본 논문 : 블라인드 텍스트 SR을 위한 생성 구조 우선순위를 내장한 최초의 시도인 MARCONet을 제안
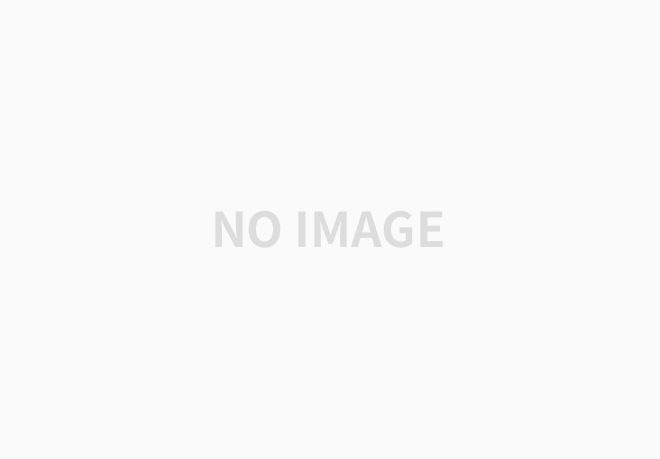
주로 세 부분으로 구성됨
1) 주어진 LR input을 기반으로 font style, character bounding boxes, code book의 index 예측
2) 각 character에 대한 structure prior 생성
3) 복원을 위한 guidance로 generative structure prior를 가지는 SR 프레임워크
[Proposed Method - 1. Pre-training of Generative Structure Prior]
추후작성